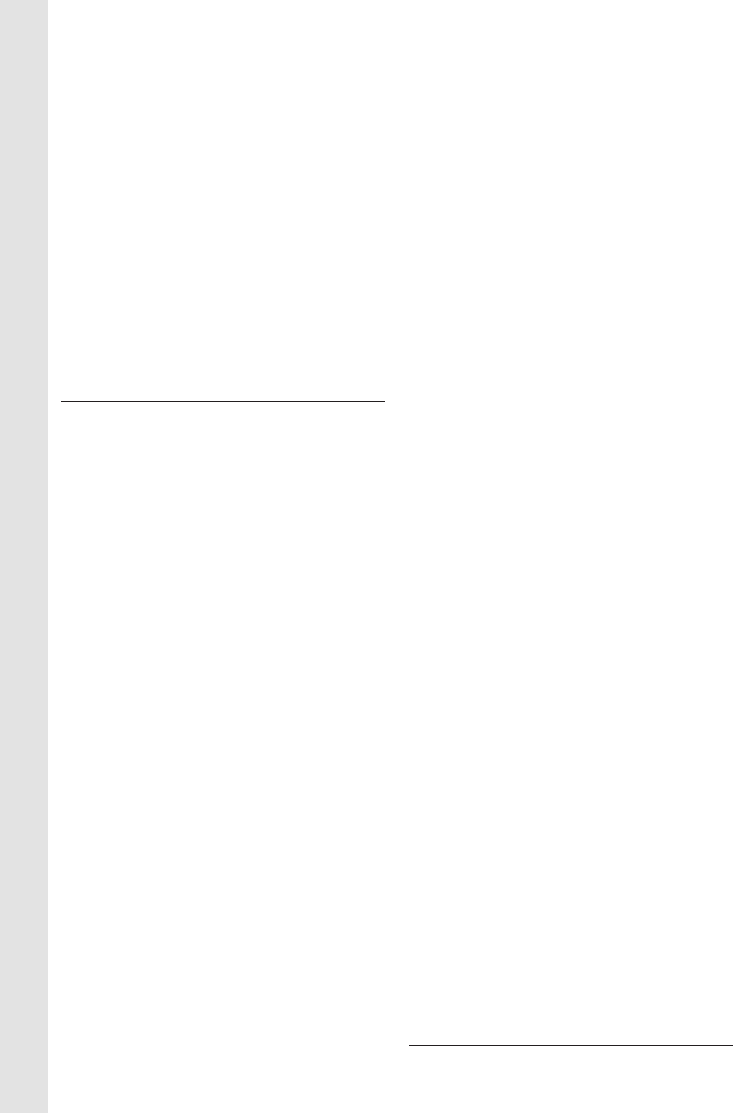
tion (3). Like Equation (2), Equation (4)
depends not only on the width of the
expected CI but also on the magnitude of
the proportion itself. Also like Equation
(2), Equation (4) does not require an in-
dependent estimate of SD because it is
calculated from p within the equation.
As an example, suppose an investigator
would like to determine the accuracy of a
diagnostic test with a 95% CI of ⫾10%.
Suppose that, on the basis of results of
preliminary studies, the estimated accu-
racy is 80%. With these assumptions,
D ⫽ 0.20, p ⫽ 0.80, and z
crit
⫽ 1.960.
Equation (4) yields a sample size of N ⫽
61. Therefore, 61 patients should be ex-
amined in the study.
MINIMIZING THE SAMPLE SIZE
Now that we understand how to calcu-
late sample size, what if the sample size
we calculate is too large to be feasibly
studied? Browner et al (16) list a number
of strategies for minimizing the sample
size. These strategies are briefly discussed
in the following paragraphs.
Use Continuous Measurements
Instead of Categories
Because a radiologic diagnosis is often
expressed in terms of a binary result, such
as the presence or absence of a disease, it
is natural to convert continuous mea-
surements into categories. For example,
the size of a lesion might be encoded as
“small” or “large.” For a sample of fixed
size, the use of the actual measurement
rather than the proportion in each cate-
gory yields more power. This is because
statistical tests that incorporate the use of
continuous values are mathematically
more powerful than those used for pro-
portions, given the same sample size.
Use More Precise Measurements
For studies in which Equation (1) or
Equation (2) applies, any way to increase
the precision (decrease the variability) of
the measurement process should be sought.
For some types of research, precision can
be increased by simply repeating the
measurement. More complex equations
are necessary for studies involving re-
peated measurements in the same indi-
viduals (17), but the basic principles are
similar.
Use Paired Measurements
Statistical tests like the paired t test are
mathematically more powerful for a
given sample size than are unpaired tests
because in paired tests, each measure-
ment is matched with its own control.
For example, instead of comparing the
average lesion size in a group of treated
patients with that in a control group,
measuring the change in lesion size in
each patient after treatment allows each
patient to serve as his or her own control
and yields more statistical power. Equa-
tion (1) can still be used in this case. D
represents the expected change in the
measurement, and is the expected SD
of this change. The additional power and
reduction in sample size are due to the SD
being smaller for changes within individ-
uals than for overall differences between
groups of individuals.
Use Unequal Group Sizes
Equations (1) and (2) involve the as-
sumption that the comparison groups are
equal in size. Although it is statistically
most efficient if the two groups are equal
in size, benefit is still gained by studying
more individuals, even if the additional
individuals all belong to one of the groups.
For example, it may be feasible to recruit
additional individuals into the control
group even if it is difficult to recruit more
individuals into the noncontrol group.
More complex equations are necessary
for calculating sample sizes when com-
paring means (13) and proportions (18)
of unequal group sizes.
Expand the Minimum Expected
Difference
Perhaps the minimum expected differ-
ence that has been specified is unneces-
sarily small, and a larger expected differ-
ence could be justified, especially if the
planned study is a preliminary one. The
results of a preliminary study could be
used to justify a more ambitious follow-up
study of a larger number of individuals
and a smaller minimum difference.
DISCUSSION
The formulation of Equations (1–4) in-
volves two statistical assumptions which
should be kept in mind when these equa-
tions are applied to a particular study. First,
it is assumed that the selection of individ-
uals is random and unbiased. The decision
to include an individual in the study can-
not depend on whether or not that indi-
vidual has the characteristic or outcome
being studied. Second, in studies in which
a mean is calculated from measurements of
individuals, the measurements are as-
sumed to be normally distributed. Both of
these assumptions are required not only by
the sample size calculation method, but
also by the statistical tests themselves (such
as the t test). The situations in which Equa-
tions (1–4) are appropriate all involve para-
metric statistics. Different methods for de-
termining sample size are required for
nonparametric statistics such as the Wil-
coxon rank sum test.
Equations for calculating sample size,
such as Equations (1) and (2), also pro-
vide a method for determining statistical
power corresponding to a given sample
size. To calculate power, solve for z
pwr
in
the equation corresponding to the design
of the study. The power can be then de-
termined by referring to Table 2. In this
way, an “observed power” can be calcu-
lated after a study has been completed,
where the observed difference is used in
place of the minimum expected differ-
ence. This calculation is known as retro-
spective power analysis and is sometimes
used to aid in the interpretation of the
statistical results of a study. However, ret-
rospective power analysis is controversial
because it can be shown that observed
power is completely determined by the P
value and therefore cannot add any ad-
ditional information to its interpretation
(19). Power calculations are most appro-
priate when they incorporate a minimum
difference that is stated prospectively.
The accuracy of sample size calcula-
tions obviously depends on the accuracy
of the estimates of the parameters used in
the calculations. Therefore, these calcula-
tions should always be considered esti-
mates of an absolute minimum. It is usu-
ally prudent for the investigator to plan
to include more than the minimum
number of individuals in a study to com-
pensate for loss during follow-up or other
causes of attrition.
Sample size is best considered early in
the planning of a study, when modifica-
tions in study design can still be made.
Attention to sample size will hopefully
result in a more meaningful study whose
results will eventually receive a high pri-
ority for publication.
References
1. Pagano M, Gauvreau K. Principles of bio-
statistics. 2nd ed. Pacific Grove, Calif:
Duxbury, 2000; 246–249, 330–331.
2. Daniel WW. Biostatistics: a foundation
for analysis in the health sciences. 7th ed.
New York, NY: Wiley, 1999; 180–185, 268 –
270.
3. Altman DG. Practical statistics for medi-
cal research. London, England: Chapman
& Hall, 1991.
4. Bond J. Power calculator. Available at:
http://calculators.stat.ucla.edu/powercalc/.
Accessed March 11, 2003.
312
䡠
Radiology
䡠
May 2003 Eng
R
adiology